About Us
MuLan-Methyl is a new a deep learning framework for predicting DNA methylation sites, which is based on multiple (five) popular transformer-based language models. The framework identifies methylation sites for three different types of DNA methylation, namely N6-adenine (6mA), N4-cytosine (4mC), and 5-hydroxymethylcytosine (5hmC). Each of the five employed language models is adapted to the task using the "pre-train and fine-tune'" paradigm. Pre-training is performed on a custom corpus consisting of DNA fragments and taxonomy lineages using self-supervised learning. Fine-tuning then aims at predicting the DNA-methylation status of each type. The five models are used to collectively predict the DNA methylation status. MuLan-Methyl performs very well on a benchmark dataset. Moreover, the model captures characteristic differences between different species that are relevant for methylation. This work demonstrates that language models can be successfully adapted to this domain of application and that joint utilisation of different language models improves model performance
.Publication:
Wenhuan Zeng, Anupam Gautam, and Daniel H. Huson. "MuLan-Methyl—multiple transformer-based language models for accurate DNA methylation prediction." GigaScience, Volume 12, 2023, giad054. (Link).
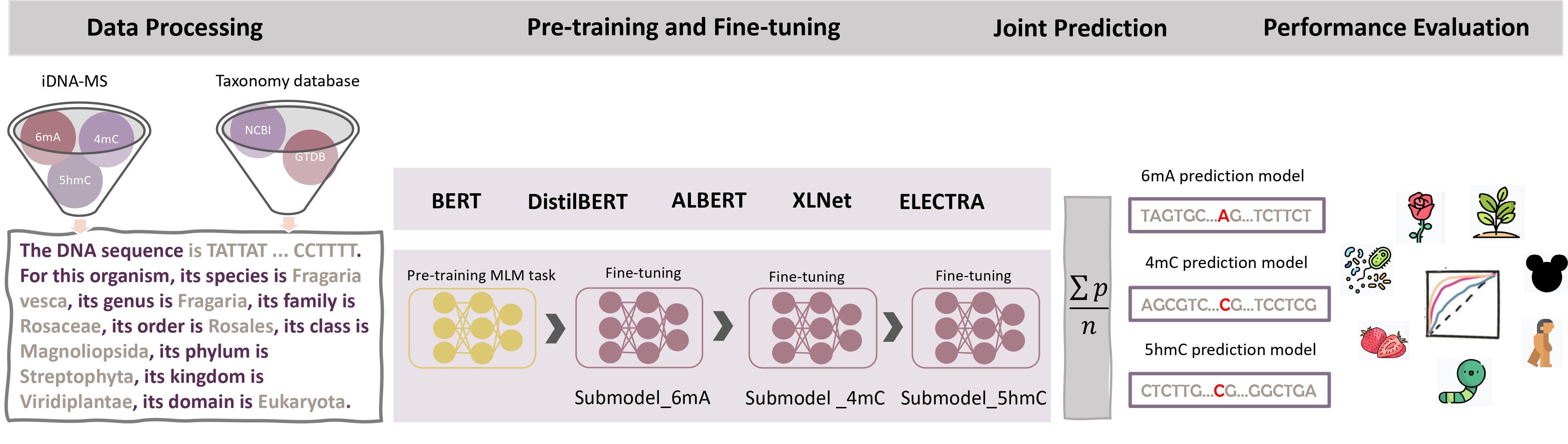
The MuLan-Methyl workflow: The framework employs five fine-tuned language models for joint identification of DNA methylation sites. Methylation datasets (obtained from iDNA-MS) are processed as sentences that describe the DNA sequence as well as the taxonomy lineage, giving rise to the processed training dataset and the processed independent set. For each transformer-based language model, a custom tokenizer is trained based on a corpus that consists of the processed training dataset and taxonomy lineage data from NCBI and GTDB. Pre-training and fine-tuning are both conducted on each methylation- site specific training subset separately. During model testing, the prediction of a sample in the processed independent test set is defined as the average prediction probability of the five fine-tuned models. We thus obtain three methylation type-wise prediction models. We evaluated the model performance according to the genome type that contained in the corresponding methylation type-wise dataset, respectively. In total, we evaluated 17 combinations of methylation types and taxonomic lineages.
The joint work, is mostly promoted by Wenhuan Zeng (University of Tübingen), Anupam Gautam (University of Tübingen & IMPRS-"From Molecules to Organisms", Max Planck Institute for Biology Tübingen), and Daniel H. Huson (University of Tübingen). We encourage all researcher around the world to contact us and to share your ideas on how to improve or extend our services.
Version History and Warranty
MuLan-Methyl v.1.02 - Mar 2023
Copyright (C) 2023-2024 WSI-ZBIT/IBMI. This web platform and all its services/tools come with ABSOLUTELY NO WARRANTY.
All services (if not restricted) are freely available for ACADEMIC NON-profit institutions, otherwise do not hesitate to contact us by email at ab-support[at]inf.uni-tuebingen.de.